What are the challenges and opportunities of AI for ResearchOps?
This is It's a Great Research Ops Question, a 6 part pod cast series produced by the ChaCha Club, a member's club for research ops professionals. In each episode, a panel of club members will tackle a great question about research ops, like what does AI mean for research, or how do you build a compelling business case for research ops? This series is sponsored by Great Question, the all in one UX research platform, and I'm your host, Kate Tauzy and the founder of the ChaCha Club and the author of Research That Scales, The Research Operations Handbook. This is episode 1. In the studio, we have Jess.
Jess Lewes:Hi. I'm Jess Lewis, research operations at Kalooza.
Kate Towsey:And Jared.
Kate Towsey:Hi. I'm Jared. I'm a research operations principal at Okta.
Kate Towsey:And last but not least, we have Tim.
Tim Toy:Hi. I'm Tim Toy, senior program manager of research operations at Adobe.
Kate Towsey:In this episode, Jared, Jess, and Tim will tackle this really great question. What are the challenges and opportunities of AI for research ops? Let's dive into the conversation. All guests views are their own and not that of their employer.
Jess Lewes:GPT is the same age as my daughter.
Kate Towsey:Ah, so it is 2 years?
Jess Lewes:November 2022, the year and
Kate Towsey:a half. Wow. Okay. It feels like a few years ago, we've been reminded, Jess, thank you, that your daughter is as old as Chat GPT, which is now what did you say? November 2022?
Kate Towsey:That's right. Great. And it feels like before then, AI was something that was in sci fi movies, in sci fi books. It was this futurist thing that might come down the line, but wasn't gonna happen anytime soon. And that, to me at least, it it felt like suddenly it sort of arrived on the scene.
Kate Towsey:And, there's so much question about how do everything seems to have some artificial intelligence piece to it now, every tool. And there's this big question, what do we do with artificial intelligence? How is you know, what are the challenges and opportunities of AI in the world of research operations? And so this is the real question we're here to kinda think and talk about today. The best place to start is what is artificial intelligence?
Kate Towsey:Jess, you have a really, you've got an interesting background with this. Do you wanna, like, in a sentence, tell me because you've done a thesis on research and AI. Right? Okay. So we've got artificial intelligence and machine learning are 2 different things.
Jess Lewes:Artificial intelligence is the broader the whole thing. But my understanding, and I'm not a technical expert, I'm an operations person, is that you can have something that is artificially intelligent without it necessarily using a machine learning algorithm, so specifically machine learning. The clue's in the name it learns, but not all AI learns necessarily. It might be that it's sort of set to do a certain thing that is smart, but it won't improve over time.
Kate Towsey:Okay. Great. So it feels like something like, with the kinds of things that we mostly are seeing in the the tools, that's machine learning artificial intelligence a la chat gpt?
Jess Lewes:Well, more deep learning. Again, this is just my very and when I did the dissertation and the the thesis, you know, you suddenly learn all the things that you don't know, and then it does become a bit scary. But the deep learning is, you know, the black box stuff, where you don't actually understand how it's got to the conclusion that it's given you. And I think where the realms where we're working within, that's perhaps more advanced than maybe what would be practically useful for us right now in in research. And we'll particularly from my perspective, I'll we'll get into that later as to why I don't think the really scary stuff is actually applicable in user research.
Kate Towsey:Really interesting. So, Jared, you had an interesting we we looking at this, how do we distinguish between artificial intelligence generally and AI that is specifically focused on research use cases?
Jared Forney:I think kind of what's interesting about this is there's this separation, right, in our heads of, like, how we experience, AI and machine learning models in our lives outside of work, potentially, and outside of a research and research operations context. And then there's the way that we're experiencing it in that context, you know, oftentimes embedded in tools or even those public facing tools being applied for research purposes. So I know, like, when in my, experience with AI and machine learning models in my day to day work, a lot of what I'm focused on is around, what would, like, broadly be considered to be, like, small language models. Right? So it's, like, these, kind of tactically applied, machine learning models, that are very focused on a very specific subset of data rather than kind of indexing just to be general, but indexing all of the Internet, all of our kind of collective experience for AI machine learning, and instead focusing on, like, really small subsets of data, like, embedded within certain tools.
Jared Forney:So I think that's, like, the first thing that comes to mind to me is, like, how we approach this might vary depending on what tools we use or what approach we take.
Tim Toy:Yeah. I think in terms of definitions, I was thinking along the lines of what is AI useful for when research use cases versus research operations use cases. Because what we see a lot is more oriented towards the act of doing user research, whereas now I'm more curious about, like, where do we define that line between what's gonna help research operations, which we're all we all are versus what's on the other side of the house. So that that's kind of one of my kinda topics I'm curious to dive in with Jess, you and Jared on.
Kate Towsey:Well, should we go straight to that? Was there anything else any of you wanted to add to that that definition piece?
Jess Lewes:No. It was really just that I think that Tim's point is a really great segue into the next part of the conversation. And I think one of the challenges that operations professionals are facing is how do we evaluate this new technology that isn't yet known. And I think starting to break that down between the tools that will help the operations side and the practicalities around facilitating a person to be in a place to enable a research thing to happen, you know, getting the the right participant in the right place at the right time versus some of the more complex potential uses of AI in actually analyzing and generating insights. And I think starting to understand how we evaluate that is is categorizing some of the the tasks.
Kate Towsey:What I was hearing there, Jess, is, you've got, there's the promise of of artificial intelligence and what it can bring. And a lot of people are hopping to that kind of future phase of it's smart enough and reliable enough that it can analyze research data and come up with really fantastic reliable insights. This is the really practical stuff of it can eliminate annoying task. Or it could, you know, sort of the middle spectrum of that, it can really help us boost recruiting by doing bits and pieces of recruiting that are, have been needed to be done manually. And so there's automations within the the, like, the workflows of getting research done as opposed to actually doing the research for us.
Kate Towsey:So let's start with, you know, are you finding it a challenge to to help researchers or your managers understand that distinction? Are they hopping into the future and weighing that piece first?
Tim Toy:Yeah. I can jump in there. I think one of the most interesting things our researchers have kind of, demonstrated is our reticence to kind of engage with the AI. We actually did a in the past kind of a working group where our researchers did an audit with publicly available data. And the thought was the analogy was really good.
Tim Toy:They just said it feels like a college age intern that I need to double check on a lot. And, they felt like there was a level of hand holding and coaching that they constantly needed to do, and they said, you know, at the current moment, you know, in 2020 middle of 2024, it's not quite there, but they acknowledge, like, things are evolving fairly quickly. So we're very curious to see what is re and I think Jess and Jericho have the same experience, like, what is real versus what is just kind of smoke and mirrors. And I think that's one thing we're trying to discern from, like, everything seems to have AI in it. Now what is actually useful?
Tim Toy:And that's that's kind of the hard part we're trying to figure out.
Jess Lewes:Particularly when it comes to evaluating a supplier we're about to renew with one of our, suppliers, that we use as a research repository, and and the potential cost increase if we go for the price plan that's got all of these great features is gonna be quite different to what we paid when we last renewed. And so I'm looking at how do we build that when, you know, I don't know really if it's gonna add the value that, you know, the sales people might say it say it adds. Because also, when I talk to my colleagues, about some of the features that are supporting the research part as opposed to the operations part. You know, the questions that I get around, for example, any AI that might enable, or facilitate some analysis is how do you know that you're gonna really, as a researcher, understand that? And I actually have been going back to the Research in UX User Research book by James Lang and Emma Howell because, you know, we all love to nerd out and read books.
Jess Lewes:Right? So I was looking at the the analysis section of that because I'm about to embark on a big piece of sort of coordinating some analysis across a number of teams. And they really emphasize the importance of just sitting in that data and absorbing it. And if we're potentially going to outsource parts of that process to some sort of AI, firstly, does that mean that we're not going to get to the real juicy insights? And again, I'm gonna quote a brilliant colleague of mine, Karen, that she talks about this moment.
Jess Lewes:And secondly, this is again an an ops challenge, is one of the parts of an ops role. It's not explicitly a part of my role, but it certainly is something that other research ops people will be responsible for is, that pillar around people and how we develop skill sets and career frameworks, etcetera etcetera. And if we've got, you know, next generation of people coming up who are really adept at the prompts that you need to put into generative AI to get it to spit out a research report that is in their tone of voice. How do you know that they haven't just skipped a bit of that and they're not really thoroughly developing these rigorous analytical skills? I feel like I've jumped right into something meaty there and sort of skipped part of the initial question.
Jared Forney:Yeah. I think just to to add just to what you're saying there and especially, Tim, like, to speaking about, like, the kind of constant moving target of these all these new processes and procedures and how we're evaluating AI and research generally. I think, for our organization, part of the challenge is, like, we're we're kind of writing the guidelines for it and establishing a baseline for what it means for our organization as the tools themselves are changing. So I know in my role as as a re ops professional, like, a big part of that has been helping to co author that by showing our legal and security teams what the landscape looks like from our end. And with that comes the challenge of it's kind of a a catch 22 where you wanna prove the value of the tool, but you can't prove the value unless you can get into an environment where you can demonstrate it with as close to real data as you can because you're trying to get approvals to be able to use those tools internally, but you can't prove the value without the approval.
Jared Forney:And so it's been kind of a balancing act of being able to show teams what what, what looks to be possible or what is possible today. And then, also emphasizing that, like, we're doing this in a very in applying it in a very intentional way. And and and I think to that end, Jess, like what you said about, the concern about having AI take away from those moments and being embedded in the data, I think is a really important point because that's, like, a really fundamental part of the research discipline is about being immersed in the voice of your customer, of your user, of the people you're serving. Right? But I think the opportunity for some of this, at least what we found in our early implementations of some of our AI tools, is giving people a an easy first rung, right, of that ladder, a really easy exposure point.
Jared Forney:And some of the tooling, that we've implemented AI for, it's come down to in search, like, really helping to address that first step problem of, like, I can't find anything searching in our repository tools that's relevant. Or, like, I just want, like, 3 bullet points to help me get up and go. And that's where, like, the AI tools have, like, started to suggest or surface insights on a on a plain English topic or a search that somebody's doing, and it entices someone to dig deeper into the data. And I think that's, at least for us in our early implementations, that's where the opportunity is. So there's a lot of challenges in, like, getting buy in and making sure we're doing things in an above board, you know, appropriate way in terms of access and and how much we're relying on that data.
Jared Forney:But on the other hand, there's a lot we're already starting to see some early opportunities for how we can implement those AI tools. I think
Jess Lewes:also not I think the the thing about AI is it makes you want to skip ahead to be like, yeah, we can write hovercrafts, and it'll do everything for us. Well, And, actually, just remembering that there were some really super simple basic things, like having, the gen generative AI powered transcripts and just throwing all of our because post COVID, all of our research at the moment is through Google Meets. They're recorded, which prior to COVID, we wouldn't have that asset. And being able to throw it in our repository and generate a transcript even before we've got to that point of analysis means that you're not constantly badgering people across the org who do research. You'd be like, did you fill in my table to say you're about to do research on a thing?
Jess Lewes:Enabling people to join up. And also, we work in a b to b setting. It's really complex, silos, etcetera, etcetera. Someone in one team might be talking to a user. A lot of our users are customer call care agents and call centers.
Jess Lewes:The session itself may be about one particular thing, but they may talk about something that's relevant to another team, and we might not know that that's relevant. But the beauty of having this very basic thing of having the transcripts being searchable now means that you can hop in, search, find it, and make those connections that maybe didn't exist. And that's not even that complex, really, in the grand scheme of what is potentially possible, but it's been really valuable for us.
Tim Toy:Yeah. With some of the implementations we've seen, we've actually similar to what you're saying, Jess. Our researchers are wanting to sit in the data. They don't want the automated summary analysis. They feel like that's their wheelhouse.
Tim Toy:But some of the things at the planning stage, at the ideation stage, our researchers have basically said it can be like a double check for you, at go to a generative AI and see if I'm asking double barreled questions or if I'm writing at a certain, grade level if I'm targeting a specific audience. So they've gotten really creative with different ways to use generative AI and still feeling like it belongs to them, and that's, like, one big thing that they really care about, which I totally understand. And they've seen really good success with, like, kind of the planning stage of whether, like, write me a screener that will find x y z, and I think that's where they want to get to and it's kind of crosses over into that research ops realm where it's, like, where do we kind of plug in? Because to your point, Jared, it is really hard to onboard any of these things because I'm sure our procurement and security teams are so overwhelmed by every other team's vendors saying we have new generative AI. So it's up to us to prove it and then we can't use the real data that we have.
Tim Toy:So we just kind of kinda get stuck. I mean, it's like a snake eating its own tail where we're just kind of stuck. So, I'm glad our researchers have at least found some ability to use the generative AI tools we have to provide ideation, inspiration, and be a little bit more creative. One of our I don't know if I let's just say the example was they were or targeting 9 through 13 year olds. They tried to make the screener a little bit more appropriate for that reading level, and it was something that I would never have thought of or know how to do.
Tim Toy:And it was one of those use cases like, oh, that's cool and we should look for more kind of unique use cases like that to figure out maybe there's other ways to use it. So we we've been having some fun. 1 of our researchers said, I want a screen that talks like a pirate just to see if it would and it did and it did really well.
Jess Lewes:There is some really fun stuff, and I think this is really the important distinction is rather than getting distracted by the shiny bells and whistles. It's about seeing, there's just a good phrase, I think, works for me in terms of how to describe this as it's, it augments your capabilities. It's not something that you can sort of ship it out ship a task out to it. It's, maybe extend extending your capabilities to some degree, but understanding the flaws in that in the way that you would. I think, Tim, in one of the things that you put on our sort of discussion guide that we shared before this, Yeah.
Jess Lewes:You mentioned already that it's almost like a research intern, that in if you had a research intern, and I'm not suggesting we should use AI to not give people an opportunity to be an intern. But with any other colleague, you know their strengths and weaknesses. We are all human beings with bias and weaknesses, etcetera, and it's the same with this technology. So, you know, if you go into it with that knowledge, I think that that example you just gave is brilliant of using it to almost bounce ideas off. And that's certainly something that's important for us because we're scaling rapidly with well, like many organizations, you know, you're trying to ship stuff out.
Jess Lewes:And, actually, there isn't always someone sat next to you if you're working remotely to go, what's a better way of phrasing this word?
Jared Forney:Yeah. I I think that there's a lot there's a lot there in that kind of partnership or, you know, and and if you all like, copilot's a word I hear used a lot to to the point where now I'm realizing it's probably already a trademark term. But the the idea of this, like, partner's copartnership of a an augmentation or extension of our abilities and the things we're doing, I think, is really also resonating a lot with a lot of re ops professionals, especially with teams of 1. Right? So many so many of us are on our own, you know, having to figure things out as we go, and we're looking for anything that can help remove repetitive tasks, tedious tasks, tasks that are really detail oriented, but at the same time, very in in really large volumes.
Jared Forney:So maybe they don't have a lot of impact by themselves, but in aggregate, they do. And it's very tedious and time consuming to do them, especially when you have 6 other things on your plate. Another, like, example of this that we're really excited about in some of our tools that's forthcoming is is, redaction. So, like, redaction is, like, obviously, a really important part of, reviewing transcripts and pulling things out, especially when they're consumed by a larger audience. But when you have an hour long transcript, I'm sure I'm sure everyone of us has had exposure to it, that's a lot of text to go through when you have an hour call times 10 or 15 calls for an average study.
Jared Forney:And that you know, when you're skimming for that, looking for things to redact, it's easy to miss things, or have to double check and catch them later. And the opportunity for a large language model or an AI to be able to recognize based on the custom dictionaries and language that you typically use within your organization to help catch those things or at least flag them saying, this looks like something you typically redact. Do you want us to cut that for you? Like, those are examples of things where it's like, ah, yes. This is something that would be really helpful for me and removing the tedium from the work that isn't necessarily the, you know, the work of of someone that's, like, dedicated in our field.
Jared Forney:It's not it's not removing anything from the analysis process, you know, the really important meaty stuff we we we do and and support as researchers and research ops folks. But it still adds a lot of value in the aggregate over time. So those are things that we look for, you know, in opportunity spaces when we're exploring AI. It's like a lot of AI driven, things, are starting at the solution and working backwards. And for us, it's a lot about going forward, you know, starting with the problems and working
Tim Toy:from there. I mean, it's kinda funny that you mentioned that, Jared, because I was thinking about who would do the redaction in the past. It would be a research intern who would just go sitting there with a little, like, pen. But you also touched on one thing I wanna dig in on is that the AI and the impact on a research ops team have won. Like, I've been thinking about this a lot because part of me is, like, oh, man.
Tim Toy:If we if AI works the way I want it to, it should allow me to be more strategic. But we all work in organizations that may have different opinions on research ops about its growth and I'm like, okay. Do research ops seem as a one stand to get be allowed to scale and grow by leveraging the AI, or are we gonna get kind of trapped into this little box that says, you're a team of 1 and you seem to be making it work with AI. You're just gonna stay where you are. And that's what I'm curious to get your opinions on like where do you think how do we think we can kind of combat that mentality that says like you now have your copilot to do whatever you want and have that little AI help you schedule and recruit and pay incentives.
Tim Toy:So you should be good and I'm curious to see how we are able to combat that as, like, kind of a a group because my hope is that we go automate all the boring stuff and then we can do more strategic high value ads but we will see him. Yeah.
Jared Forney:Yeah. I I think that last point is really important, Tim. Right? It's like, this is this the AI isn't replacing or or is or serving as a substitute for a headcount. Right?
Jared Forney:It's more about, like, I am delegating this set of, let's call them bucketed administrative tasks, like the repetitive things. I am delegating that now to a tool. Look what this is opening up. Look at all the strategic opportunities that are now opening up because I'm not spending 15, 20% of my time doing these other things. So it's always in service of, like, opening up the opportunities.
Jared Forney:Look how much more we could be doing now that we're able to delegate a certain part. So it's always like a yes and sort of thing rather than this, like, substitution for the work that we're doing. So I think that's, like, one thing that I'm always, like, careful to frame when we roll out new features. I think the light bulb moment for one of our stakeholders when we rolled out, like, that search example I talked about earlier was it was a member of our team that was maybe a little bit skeptical about, like, the value of our repository in the aggregate. And but then once I demonstrated, it's like, well, this now, you can query it in plain English.
Jared Forney:And I've had an opportunity just to somebody asked a research question in Slack, you know, a what do we know about x question, like, the age old classic question that anyone asks about research. What do we know about this topic? And one of our researchers' time is like, well, I kinda know a little bit about this. Let me get back to you. I can look into it.
Jared Forney:I was like, this is a perfect opportunity. Ran the search, delivered bullet points, dropped it in the channel saying, like, if you wanna find out more about this, here's a link to it. And the stakeholder came in, like, how did you do that? Like, where where has this been? And I'm like, this is the this is what the advancements that we're talking about.
Jared Forney:This is just free up our researchers to be able to quickly answer these kind of questions so that they could focus on the really deep, gnarly, generative work that we all want, you know, we all wanna focus on the aggregate.
Kate Towsey:Let's take a super short break to hear from Ned Dwyer, the co founder and CEO of Great Question. He'll share his thoughts about the topic, and we'll rejoin the panel straight after.
Ned Dwyer:There are so many opportunities for research ops in the world of AI, and it feels like we've barely scratched the surface. There's all the, like, table stakes stuff, things like, you know, automatically summarize an interview or a series of interviews, including breaking it down into chapters and defining main themes and next steps. Make it traceable. See the individual clips or highlight reels or interviews that when informed that that output or generate everything from interview guides to surveys or even studies from scratch. It's It's gonna save you a ton of time.
Ned Dwyer:It's gonna save your team a ton of time, but it's also gonna help you empower people who do research to be able to more easily self-service, whether it's knowledge or study creation. The big one I think about is data privacy and governance. How do you ensure that you're automatically redacting PII from audio and video to reduce your security risks? How are you, reducing bias by limiting who can access these models? Maybe it's only trained professionals.
Ned Dwyer:And all this can be done today. Great question. Look. And it's not without its challenges. I think we should be aware of that, but I'm confident a lot of this is gonna fade out into the distance over time.
Ned Dwyer:1st is cost and speed. Speed has definitely improved a lot, but cost has improved massively. It's come down to a fraction of what it was even 12 months ago, and that's continued to trend in that direction, which is great. You shouldn't have to pay an arm and a leg to access AI features and the products that you use and love. The second is context windows.
Ned Dwyer:How much data can you recently store and access across these models? This has also gone up a lot. It used to be you could only really do, summarization across an individual interview. Now it's easy to do across an entire study or even an entire repository. And the third and and definitely the most important is quality of the output.
Ned Dwyer:In the last 3 months, we've seen models improve from the equivalent of grade school maths to graduate level reasoning. So it's not hard to predict that they'll continue to improve beyond this over time. I've really encouraged all research ops professionals to stay super close to the world of AI, learn what's going on, and how things are evolving. It's gonna massively streamline your work over the course of the next 6 to 12 months, allowing you to spend more time on strategic initiatives across the business. It's a game changer.
Ned Dwyer:Come and check out what we're building in our approach to AI at great question .co.
Kate Towsey:The piece that I felt that we haven't spoken about yet is that very beginning bit. Are there things that you as operations people have found with AI that you're like, oh, wow. Like, recruiting is so much easier or this workflow is so much easier. Are there examples that you can give of that?
Jared Forney:I think I could speak, like, a little bit to, like, the opportunity spaces that we're starting to see about the the connective tissue between insights. I think, Jess, you spoke a little bit about this in the beginning about, like, being able to connect themes together, across disparate studies. That's something that, like, is one of the core tenets and promises of a lot of research organization tools. And in the time, I've used a couple different platforms now. And the one thing that's always in common is they make it sound so easy on paper.
Jared Forney:And the reality is is it's it's really difficult, especially when researchers and research ops people were all under more time pressures than ever with the resourcing that we have. And so a lot of times, you have just enough time to get your insights and your deliverables to the peep the stakeholders who are asking for them. And you have that kind of flash in the pan research where it's, like, immediately useful in that context. And it gets filed away for a rainy day. But there's not necessarily a follow-up step that always happens where those those studies are connected across across disciplines or across, pillars.
Jared Forney:And maybe it happens at the at the quarterly level, but really it should be a continuous process. Right? And that's what I think we're most excited about for AI being able to start to suggest things just to give a nudge saying, hey. Like, you got some insights over here in study a and some over here in study q. They seem to be related.
Jared Forney:Like, do you want us to kinda help, like, maybe start gathering some of these things together? And it's it solves that blank page problem of people being able to connect insights together. And then it's just, like, a matter of just doing the final stitching together and smoothing things out and making it in a readable format. But, like, really helping to get past that blank page problem for connecting insights, I think, what we're most excited about.
Jess Lewes:It's certainly not just the blank page. It's also just recognizing we're humans. And if you're doing a continuous research piece over a long period of time, you can't hold all that information in your head. And this is what we know machines are good at. They're good at processing large amounts of data, so let's leverage that.
Jess Lewes:I suppose to talk about the opportunity space on the sort of operations practical side of things. So the thesis I wrote as part of my master's was focusing specifically on the recruitment part. And we know that you can write models that do prediction. And one of the things that's often important when you're researching is to get your different segments, maybe people who have just done a thing, people who are about to do a thing. For Kaluza, one of the bits of tech that we do is, you can tell I'm not in a product team, bits of tech, is around supporting EV drivers with smart charging and connecting their device and utilizing the battery storage to make sure that they're charging at a time when actually in some countries, I think Australia, for example, they can give you energy for free because they've got so much solar power in Australia.
Jess Lewes:Not something that you can do in the UK, sadly, because we only get about an hour's worth of sun, apparently, in the whole of the month of June. So the dissertation really dug into how can we maybe utilize, some sort of artificial intelligence to spot patterns in responses from participants? So imagine you've got a massive insight panel in your organization of customers. You gathered a certain amount of information from them. Maybe they've responded to previous screener questions rather than manually going through all of that, picking out data points where you go, well, we know that people who are about to buy an EV typically do x, y, zed first.
Jess Lewes:Let's find a group of those people that meet those markers. And then you could be more targeted and say, we're about to do some research. Let us know if you're interested. Answer these few questions because humans are inherently bad at predicting their own behavior. But if we can combine the human with the machine prediction, we're more likely to get people who genuinely are about to go on that journey, and maybe it will help with the accuracy of our research.
Jess Lewes:So, again, it's about using the AI and the, you know, machine learning and that sort of thing at exactly the right points to maximize efficiency.
Tim Toy:Yeah. And I I those are definitely both use cases that are very aspirational for us. I would say one of the hardest parts for us as a small research operations team serving a large research team is figuring out when to carve the space to kind of invest in our own infrastructure and figure out which of these tools are actually right for our team. Jared, you brought up the team of 1 earlier and there's so many teams of ones out there that I'm it feels like you need to take extra work to get this AI in place because you are the team of 1 fielding 20 requests from all these different spaces. So it's like, how do you funnel it down or whittle it down to the thing that's gonna be the most useful for you?
Tim Toy:And I'm curious if you 2 have any strategies on how you figured it out. I would say where I work, we kind of don't we've been told what we can use, so it it does simplify for us a little bit. But I'm curious as more and more players are entering the space. How do we figure out how to kind of build the ship while it's, you know, sailing? I was on a cruise.
Tim Toy:Sorry. So many many cruise analogies are on my mind. But that's the thing I've been thinking about because I'm like, I want to try out these new functionalities, but I don't have the time and the space to really experiment and play and figure out if this is right for us.
Jared Forney:Yeah. I think this this definitely hits close to home, Tim. Tim, I definitely know what it's like from a especially from a procurement standpoint, that is, you know, working for a security company at Okta. Right? Obviously, it's first and foremost for us.
Jared Forney:And so, typically, like, our procurement cycles for things and new tools for net new tools could be 3, 4 months. And then you might add another month or 2 for specific use cases. So you're easily looking at maybe up to half a year in some cases for really deep level integration things with AI, infrastructure. And so you have to be very cognizant about what bets you're placing. Right?
Jared Forney:Because you're you're you're investing all your time that could be spent on another initiative, maybe one that's already established, maybe exploring AI in a in, in a tool that you've already procured, right, in in lieu of something that's, net new. And I think, strategically, one thing that I found really helpful is, maybe maybe kind of obvious, but just really sitting down for FaceTime with your stakeholders, the you know, all your legal teams, your privacy and product teams, your security teams. And just like, a, letting them know you're there, like, that you're the point person, and, b, just coming with, a perspective of, like, not only are you trying to demonstrate the purpose of this tool, but you're you're really framing the conversation of, like, how it fits into product research. Right? Because some of these groups aren't exposed to product or product research, UX on a regular basis.
Jared Forney:So some of it is just level setting. It's like, hi. I work with the UX research team. Here's what we do. And even just taking that 5, 10 minutes for that, like, high level before you go into the details of the functionality and how it fits into the larger picture, I found has gone, like, a really long way into just just like with a participant or or anyone in research, you're building rapport.
Jared Forney:Right? You're building trust with your within your teams and just fostering good communication that you're doing this in everyone's best interest and that you're cognizant of the challenges not only that you face on your teams with implementing this stuff, but also from their perspective and all the things that they're thinking about when it comes to rolling out new AI tooling.
Tim Toy:Yeah. You hit me. I hit so close to home about the 6 month time span. And it's interesting, like, we were talking earlier. What was your ideal AI feature 6 months ago maybe have evolved into something that you have no idea what it is.
Tim Toy:Like, it's very interesting because it does feel like we're trying to hit a moving target and I think Jess brought that up earlier. It's very difficult to know where you should place your bets. And And I think that's one of the things that I'm struggling the most with right now because there is a lot of noise and there is a lot of dirt renewals. Everyone's like, check this out. It can do whatever you want it to.
Tim Toy:And then, you know, we've all purchased something and it's been like, oh, that's not the thing that I wanted. And I think it's just really, to your point, really hard to kind of, like, weed through that kind of noise right now.
Kate Towsey:It feels like there is a need for, it's that it's that it's that most valuable resources time to be able to step back. But then also right at the beginning you all spoke about, it's not just the time, but also you need to get it through procurement, it needs to be real data, and then it costs more money. So there are these hurdles to actually just trying it out and playing and experimenting with it. And so it just takes longer to try and figure out which parts work and which parts don't, and how you use them in your particular context. I wanted to Jess, do you wanna hop in there?
Jess Lewes:Yeah. Well, there's just another sort of point, I suppose, that's important to think about is the also the impact on the process. It it will always take time to embed a new tool, and again, something that I came across in in the research that I did was that the maximum innovation is gained not just from the technology, but from the process change, from an efficiency and optimization perspective. And any kind of change in an organization takes time.
Tim Toy:Yeah. That was actually one of our guiding principles during our evaluation is that it didn't it the tool cannot disrupt the current process. Because if it did disrupt the current process, we were not gonna engage because it would just cause too much change and that is something we kind of thought about at best front because everything can shift so quickly. So we're just like, you know what? What we're doing is right now is working.
Tim Toy:Let's not augment it for some flash in the pan, basically.
Kate Towsey:I love that idea because it sounds like, one of the top tips that that that might come out of this as well. I mean, there's just so much in here. But one of them could be have a set of principles for not just the security and legal principles, but how are you actually going to think about AI within your operations? Is that something, Tim, you've mentioned that you've had a principle? Is it something that anyone else has done to think through a list of principles?
Jess Lewes:It's not something that we've got to a point of writing that out, but it's something that I was thinking about when preparing for this, about which are the bits that we are willing to open up to, you know, some kind of AI intervention versus which are the bits that are kind of almost sacred. But right now, it's it's just not right. And I I read somewhere, it might be old information. Maybe I'll just do a quick Google when the next person is talking. But, I think Italy just said no one should use chat tpt.
Jess Lewes:I'm just gonna that was in something that our Invoice Echo data protection team wrote last year, on our in on our internal confluence page. And I was like, I need to check that out. That can't be like, I'm gonna Google it now.
Kate Towsey:Yeah. I'm watching Time. We're just about on time. We've got about 7 it's amazing. There's so much to talk about.
Kate Towsey:One of the things that we haven't gotten to yet, and it might be that we can cover this, reasonably quickly, is ethics and privacy. The kind of question about, like, what are your teams, your legal, your privacy, is your security teams asking about the most? And are there any other ethical things that are popping up that you're just going like, legal don't care about this, but this is like creepy. So for instance, Tim, I mentioned that that platform that we're using, I can I can go in afterwards and and I could edit your voice, which is an amazing feature to have, but it's cool and creepy? Is anything you're seeing like that in in AI at the moment in research?
Kate Towsey:Nobody? I
Jess Lewes:wouldn't say there's something creepy, but the one thing I just want to sorry. Was someone else talking then?
Kate Towsey:No. You go for it, Jess.
Jess Lewes:Okay. Our company name is Kalusa. In transcripts, it regularly says, I am a loser. It will it take over the world? If it and we've put in you have the opportunity to put in custom vocab, and we've got the company name in there to help the AI learn that that's the company name.
Jess Lewes:But it still gets it wrong regularly.
Jared Forney:Oh, that's so that's Jaz, I can relate to that so much because the number of times I've had to go back into a transcript and correct Okra for Okta and, for for, an industry term SAML, it's a markup language. I've I've had to do salmon. So I've definitely experienced we're not we're not quite at the AI singularity yet if we're still struggling with transcripts. But, I think, like, to on a more serious note, to, like, to, like, highlight the ethical concerns. I think it's something that, really, it comes down to we're we're definitely, as a company, setting, like, high level, you know, outlines of, and guidelines and principles for the use of AI across the company.
Jared Forney:But I think, 1st and foremost, it just really comes down to, from a research operations perspective, about just having good data privacy fundamentals to begin with. Right? Like, that that comes from, like, setting all the expectations from the participant. It's around how you're managing your data workflow, how you're responding to, data subject requests, and all those fundamental workflows that existed pre AI becoming a main thing are really just the AI as a layer on top of it that it it goes deeper in some in some cases. But by really focusing on the fundamentals and and that minimum viable data collection to get only collecting only what you absolutely need to do the work and then being able to have a clear policy and procedure for how you delete it, how you respond to requests for it, how it's used and implemented.
Jared Forney:Those are all the things that I think we've just been building on those fundamentals as the new AI functionality comes in and just being really mindful about that, and that's that's set us up well so far.
Tim Toy:Yeah. I would agree with Jared on that word. If you stick to the fundamentals, if you're not putting PII into a platform, the AI can't read the PII. And then that's basically, like, we we kinda really hammer home these, like, hygiene kind of factors, like, don't if if you have PII anywhere, you shouldn't. So let's fix that.
Tim Toy:So I think there's a lot of that just, like, making sure that folks understand what isn't is not okay and what can you can and can you not feed into generative AI? And and just really making sure because, like, it's kinda funny how we're talking about the singularity, Jared. You know, there is human error, and it's like, we do have to account for that, and it'll happen, but we try to minimize it as much as possible and then hopefully sticking to those fundamentals is how you get there.
Jess Lewes:The, just for anyone that might be listening that's in the EU or UK, the GDPR or UK GDPR. There's stuff in there around making sure that if you're profiling using AI, that you I think it's that you need to get explicit consent. And I was looking on the ICO website because the information commissioner's office is just, like, the best place to go for guidance on all of this. So if you do use AI for doing any kind of profiling, so just going back to the example I gave earlier of, potentially using AI within your recruitment process to try and segment your customer base to make recruitment quicker and and more efficient, is if you use profiling to generate new information about a user, that is then new personally identifiable data And has the person does the person that you're asking consent for, do they understand really what they're consenting to? Are they gonna be aware of this new information that you're generating?
Jess Lewes:How will they get access to it. I think in the realms of user research, I'd like to think that, you know, as a bunch of professionals, we care deeply about ethics. But, certainly, when it comes to deciding whether to use this type of technology, one of the considerations certainly needs to be what's your ethical stance as an organization, and how do you apply that when you're picking a new tool and deciding how you'll use it.
Kate Towsey:Which goes right back to that notion of a set of principles. I kind of feel at some point and I I catch myself getting excited about things all the time and cooking up a ton of work for myself. But, is, you know, as a crew, as as the ChaCha Club, can we come up with a standard set of principles? What are the things that you think about, when you're implementing AI in a research environment? Jess, just pointing to the article that you've referenced here that, access to chat gpt chatbot has been restored in Italy, but it was banned by the Italian data protection authority at the start of April of a privacy concern.
Kate Towsey:So there you go. Your memory is
Jess Lewes:So, yeah, in 20 2023. So 23. Perhaps a little bit dated, but it's just interesting that that was the initial reaction was, let's just ban it. Yeah. So, yeah.
Jess Lewes:I think another, ethical point that I can't not mention because of what Kalusa does as an organization is the environmental impact of all the additional data processing. I've got a good fact actually if you allow me to just scan my Miro board. I don't know exactly when this was, but the National Grid, which is the public sector organization that I should know what the National Grid is. But they're the the organization that kind of control and demand in response of our energy systems in the UK, the chief executive said that the boom in artificial intelligence and quantum computing would drive a spike in energy demand with power consumed by data sensors surging 6 fold in 10 years, and bold action is needed to ensure demand can be met. And we had a really great talk at a local service design meetup in Bristol by someone called Ned Gartside, who works for DEFRA, and he was talking about some of this stuff and actually balancing off, the trade off of the environmental impact and the carbon that might be emitted by using it against the potential efficiency gains that you've got.
Jess Lewes:So again, just using that to factor in actually, is it worth it? Because you can do really fun stuff, like, oh, no. Let's just, you know, make this script sound like a pirate. And we've all done it. We've all played with chat GPT.
Jess Lewes:Like, oh, let's use it to coauthor a a fun thing. But I think, you know, we're maybe all running out of steam with the fun thing.
Kate Towsey:A big thanks to our guests for sharing their time and expertise and to our sponsor, Great Question. Learn more about Great Question at great question dotc0forward/ ChaCha. That's c h a c h a. In 2 weeks, a different crew of research ops professionals will tackle another Great Question. So make sure to subscribe and tune in.
Kate Towsey:This podcast is a limited edition series produced by the Cha Cha Club. We're a member's club for research ops professionals. You can find out more at chacha . club.
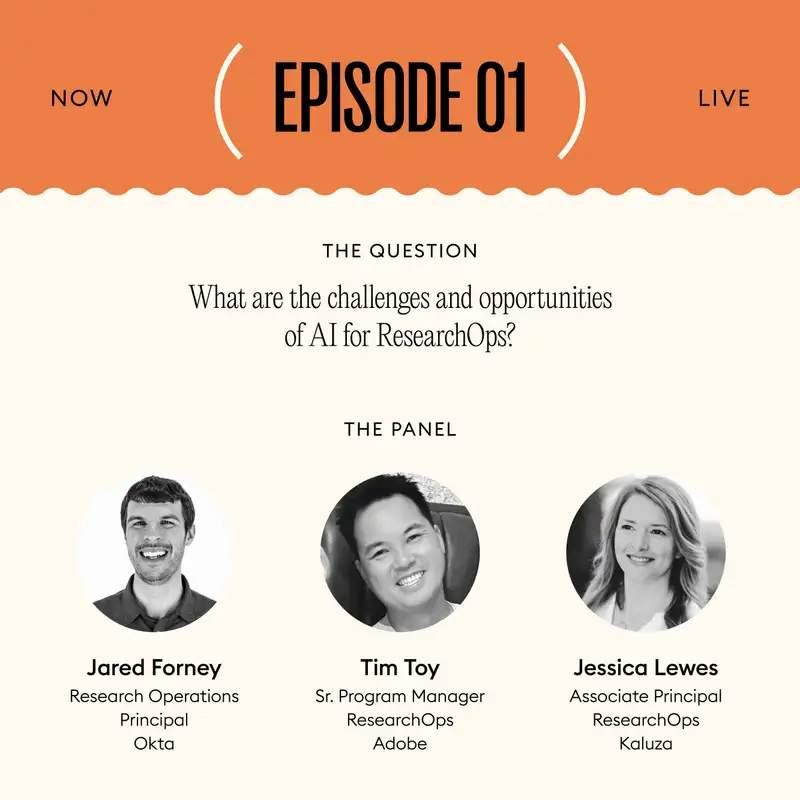